60 minutes
Time to train an inspection recipe
90 units
Inspected per minute at maximum speed
99% accuracy
Detection accuracy for particulate and cosmetic defects
Visual inspection for challenging
products
60 minutes
Time to train an inspection recipe
90 units
Inspected per minute at maximum speed
99% accuracy
Detection accuracy for particulate and cosmetic defects

AI-based inspection built for difficult-to-inspect products
Unlike traditional approaches to AI-based visual inspection, the DAI-50 uses an understanding of normal to detect the abnormal.
Powered by Boon Logic’s AVIS platform, DAI-50 can create an inspection recipe for any product in minutes, without needing a predefined set of defects. Model training requires only 500 acceptable product units.
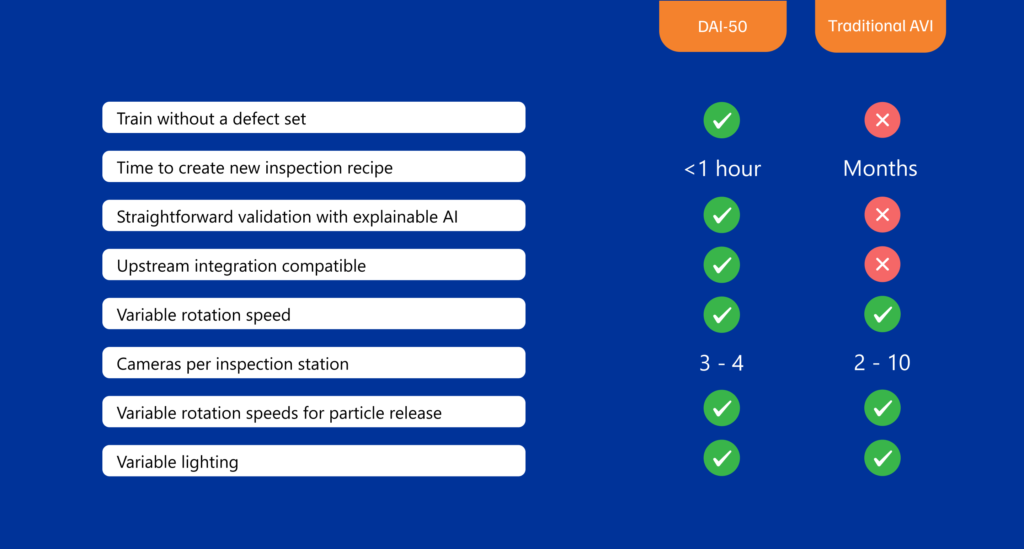
DAI-50
Traditional AVI
Train without a defect set


Time to create new inspection recipe
<1 hour
Months
Straightforward validation with explainable AI


Upstream integration compatible


Variable rotation speed


Cameras per inspection station
3 - 4
2 - 10
Variable rotation speeds for particle release


Variable lighting


Created for difficult-to-inspect products
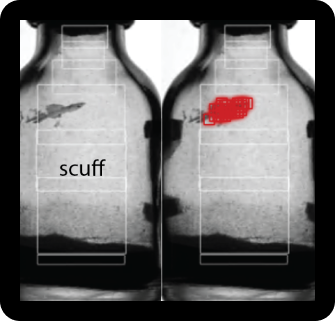
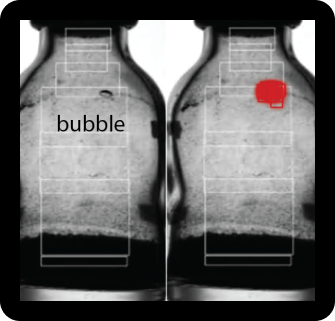
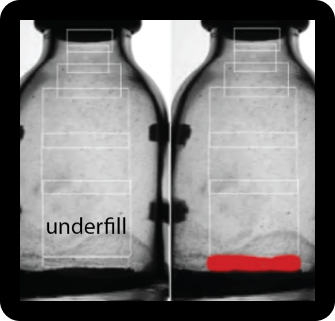
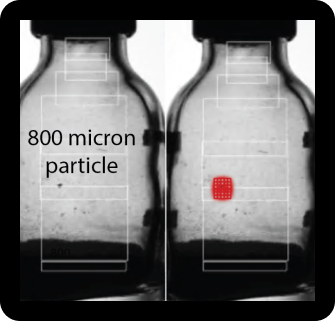
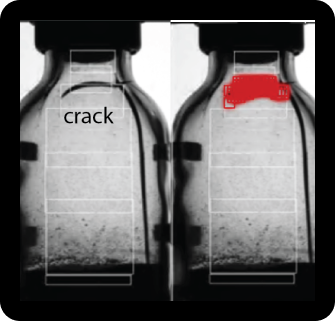
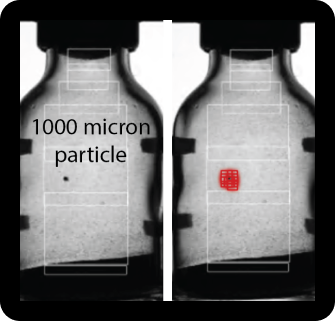
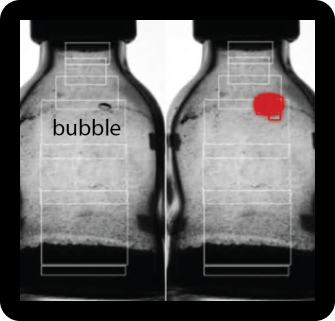
Powered by AVIS, a Boon Logic product
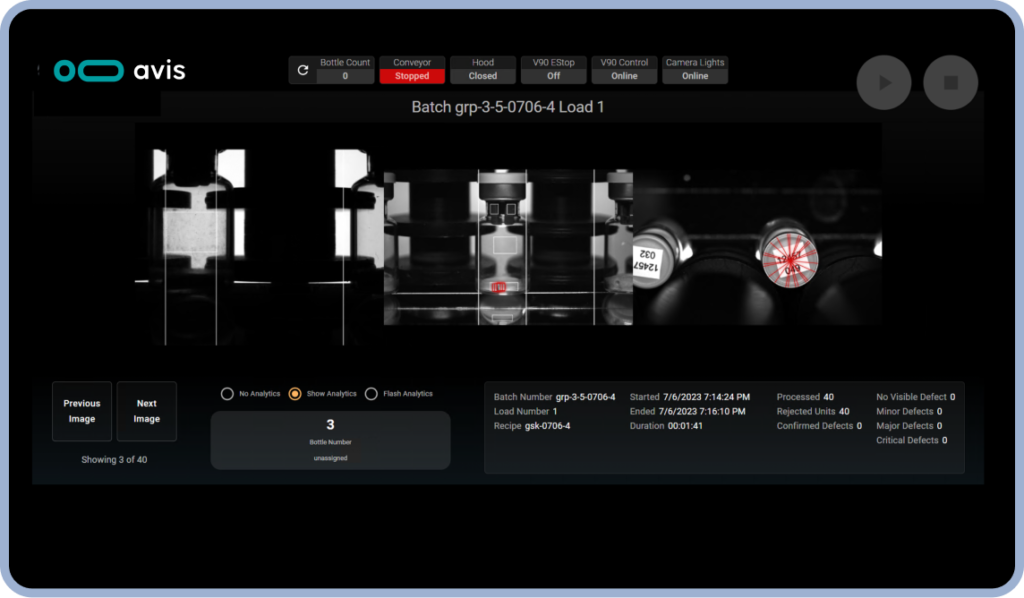
Improve the quality, consistency, and overall efficiency of your operations. AVIS automatically identifies defective products with greater accuracy than human inspectors, ensuring confidence in the quality of every unit shipped.
Detecting anomalies becomes simple once all normal variations are captured. AVIS trains on compliant products rather than relying on labeled defect images, making it the most practical solution for automating visual inspection of even the most challenging products.
- Record
- Configure
- Train
- Run
The first step in creating a new inspection recipe in AVIS is to record 500 pre-inspected compliant units, covering normal product variations like powder disposition, bubbles, fill level, glass molding, and more. AVIS uses three cameras, capturing hundreds of images from the top, inside, and outside of each unit at 30 frames per second per camera.
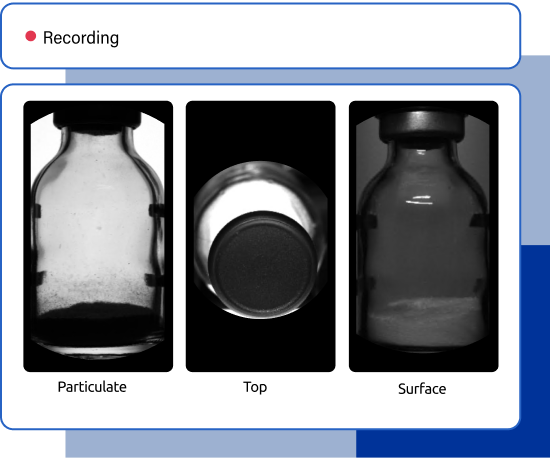
After recording, configure regions of interest (ROIs) for all three cameras. Since the units rotate 360 degrees, ROIs can be positioned in areas prone to defects, including easy-to-inspect features like the side wall and shoulder, as well as challenging areas like the vial base, oblique views of lyo cakes and powder, and tamper-evidence seals.
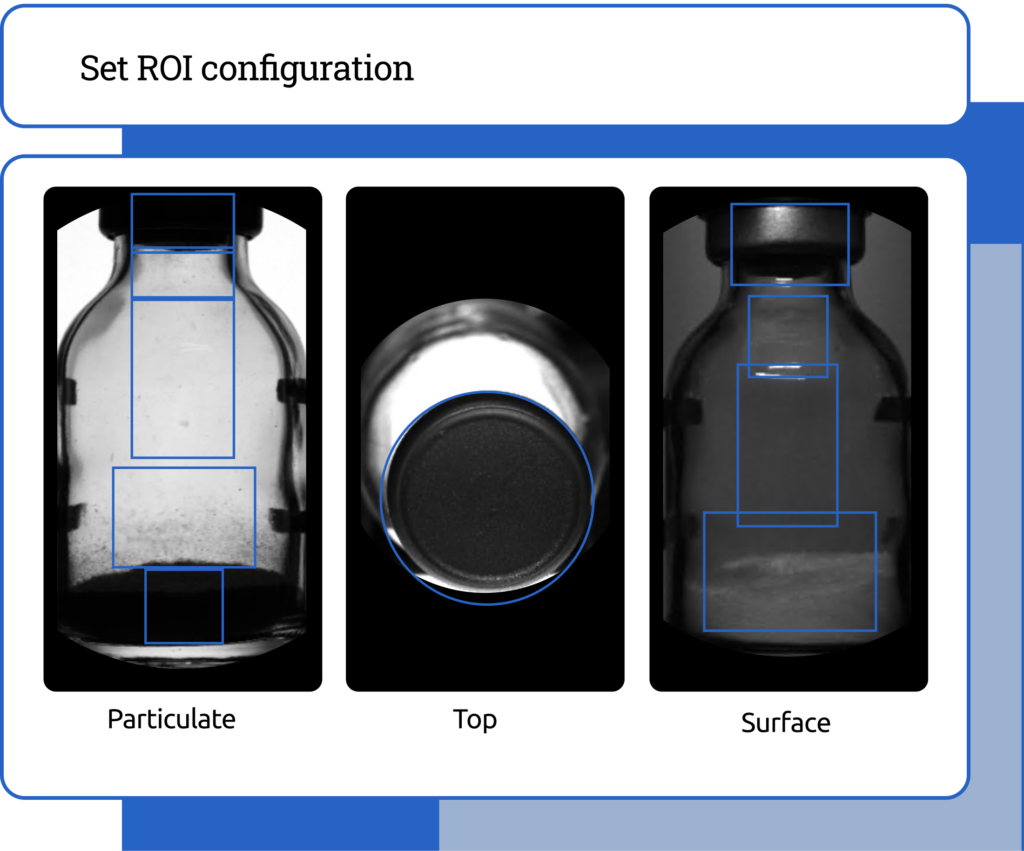
AVIS uses Boon Logic’s unsupervised machine learning algorithm, Boon Nano, to learn the normal variation for each Region of Interest (ROI). A learning curve tracks the model’s progress for each ROI. Initially, the curve is steep as AVIS learns significant variations, but it levels off toward the end, indicating that most normal variations have been integrated into the recipe. Ten unique models are trained, one for each of the 10 ROIs.
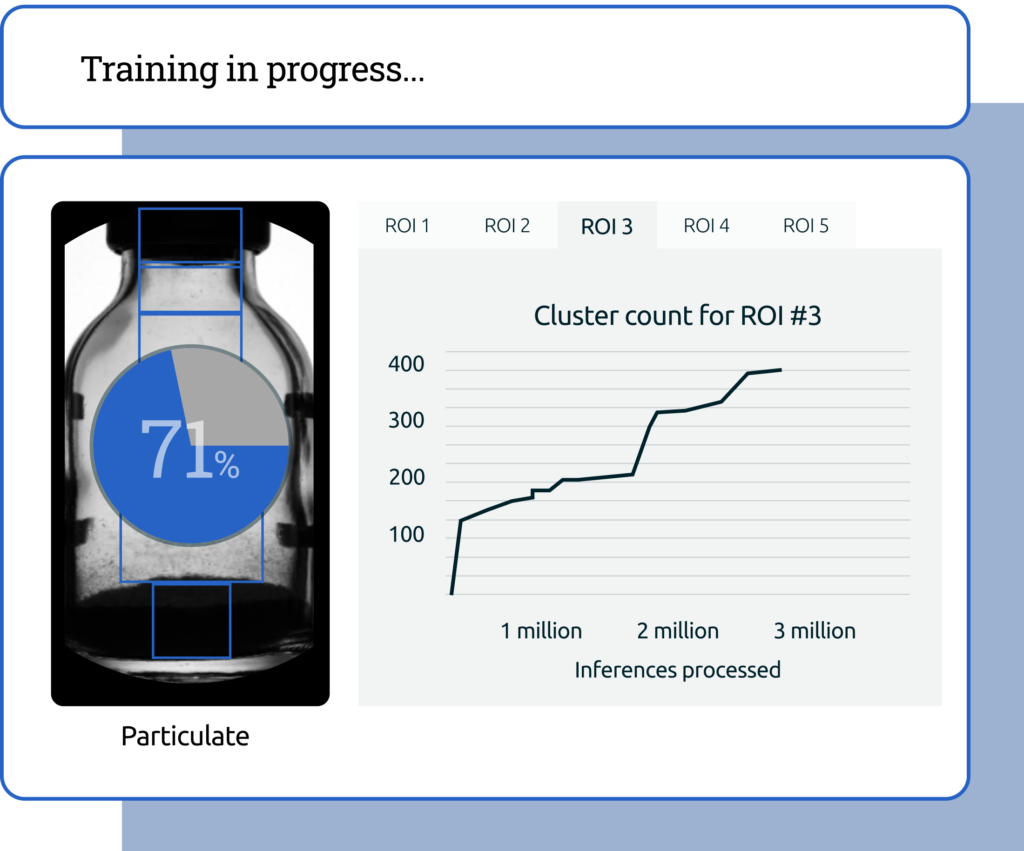
In run mode, AVIS makes 100,000 machine learning inferences per vial. Anomalies outside the learned normal variation trigger a spike in the anomaly index, indicating a potential defect. AVIS determines whether a unit is defective within milliseconds, triggering actions like ejecting the unit, stopping the conveyor, or flashing a light. The defect’s video frame, with the exact location highlighted, is displayed and archived for trend analysis.
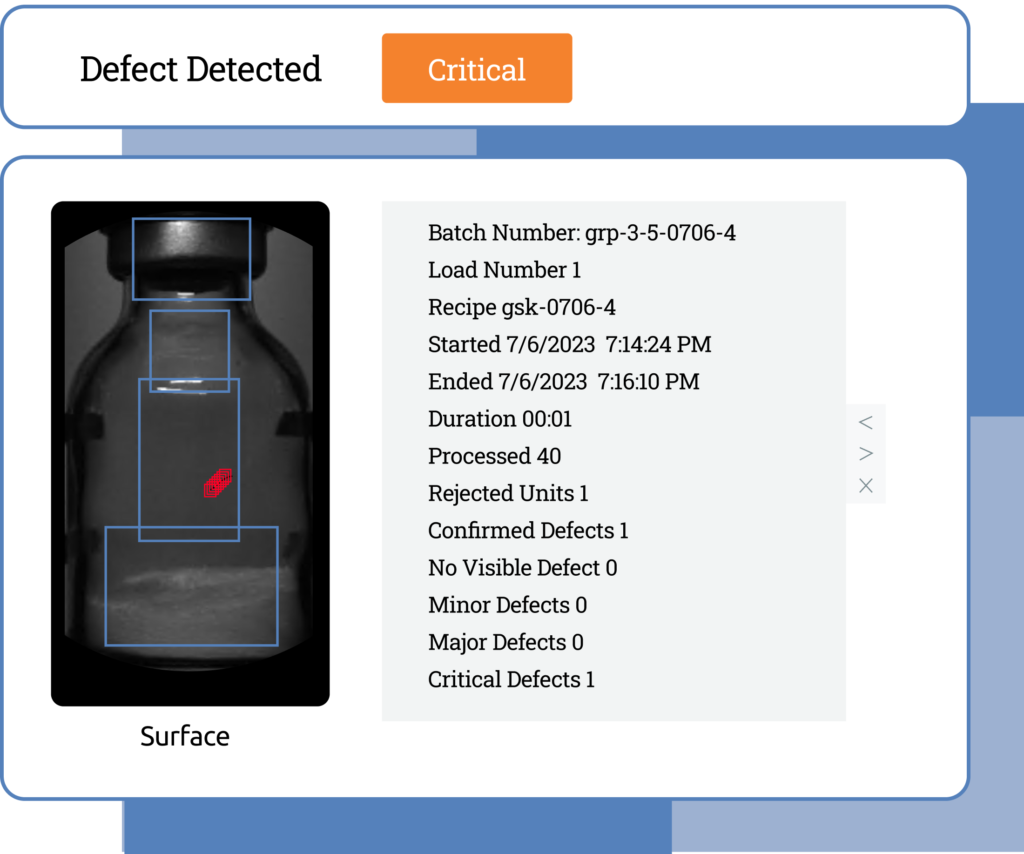
The first step in creating a new inspection recipe in AVIS is to record 500 pre-inspected compliant units, covering normal product variations like powder disposition, bubbles, fill level, glass molding, and more. AVIS uses three cameras, capturing hundreds of images from the top, inside, and outside of each unit at 30 frames per second per camera.
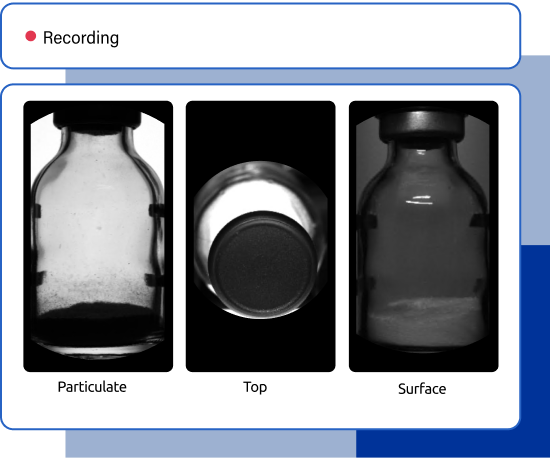
After recording, configure regions of interest (ROIs) for all three cameras. Since the units rotate 360 degrees, ROIs can be positioned in areas prone to defects, including easy-to-inspect features like the side wall and shoulder, as well as challenging areas like the vial base, oblique views of lyo cakes and powder, and tamper-evidence seals.
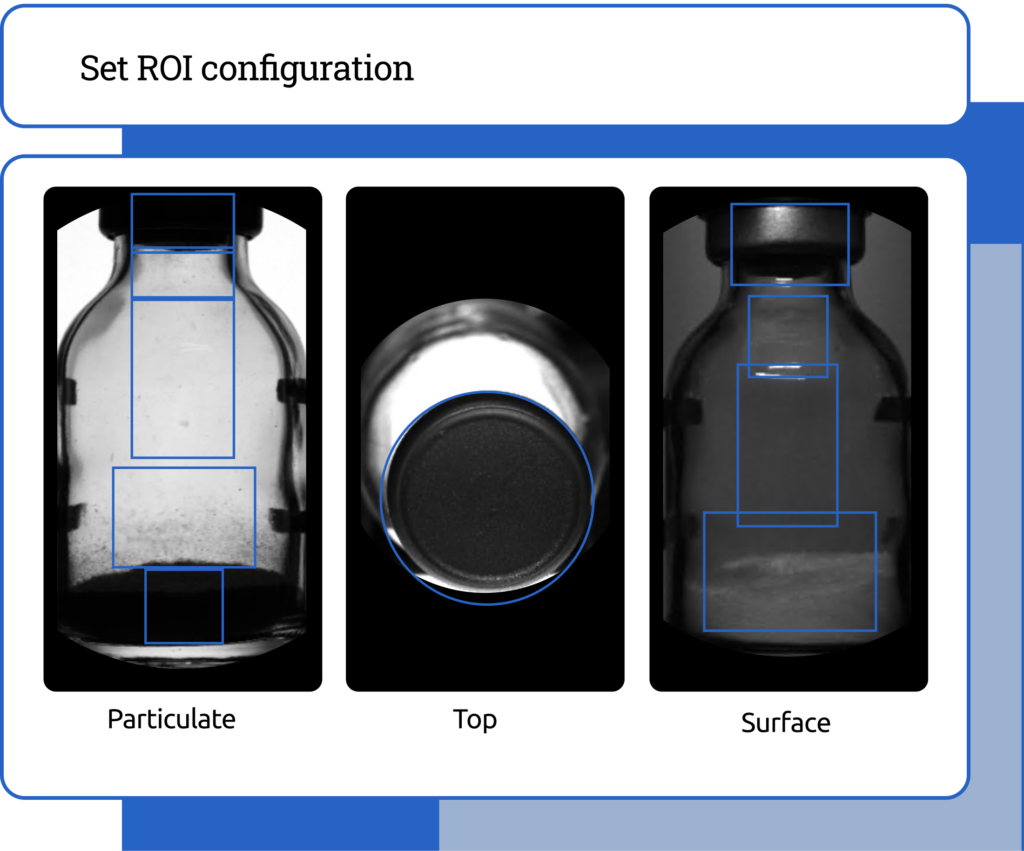
AVIS uses Boon Logic’s unsupervised machine learning algorithm, Boon Nano, to learn the normal variation for each Region of Interest (ROI). A learning curve tracks the model’s progress for each ROI. Initially, the curve is steep as AVIS learns significant variations, but it levels off toward the end, indicating that most normal variations have been integrated into the recipe. Ten unique models are trained, one for each of the 10 ROIs.
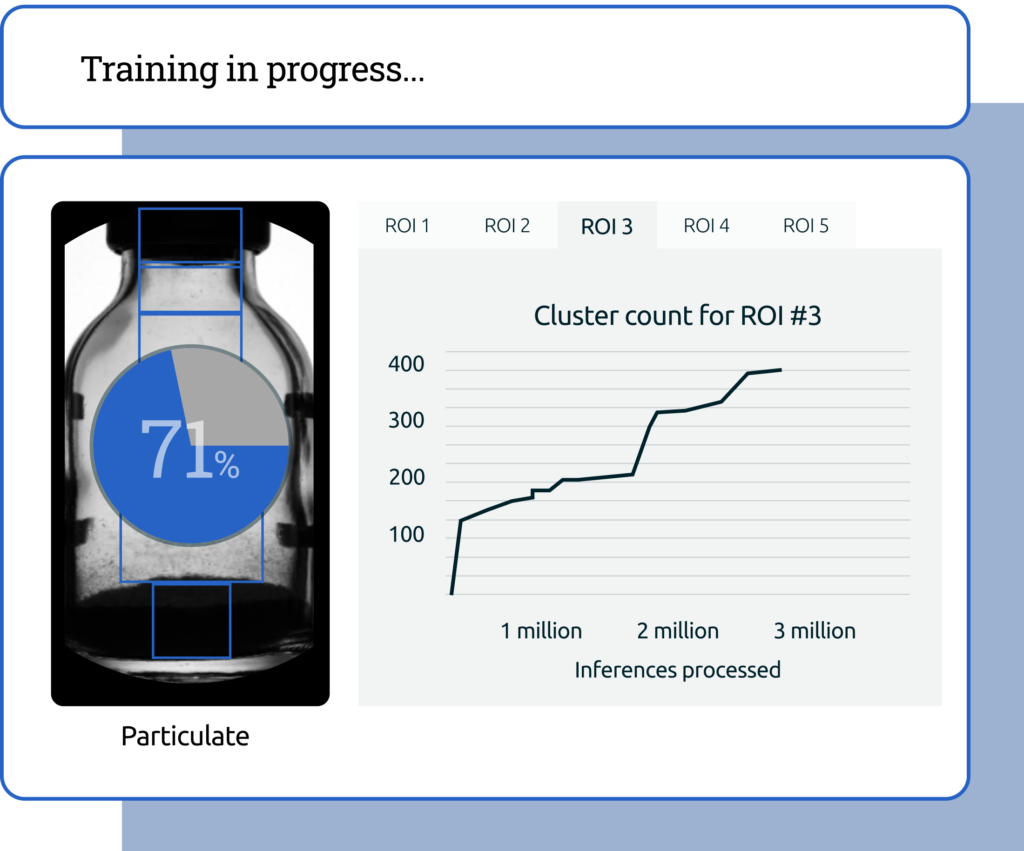
In run mode, AVIS makes 100,000 machine learning inferences per vial. Anomalies outside the learned normal variation trigger a spike in the anomaly index, indicating a potential defect. AVIS determines whether a unit is defective within milliseconds, triggering actions like ejecting the unit, stopping the conveyor, or flashing a light. The defect’s video frame, with the exact location highlighted, is displayed and archived for trend analysis.
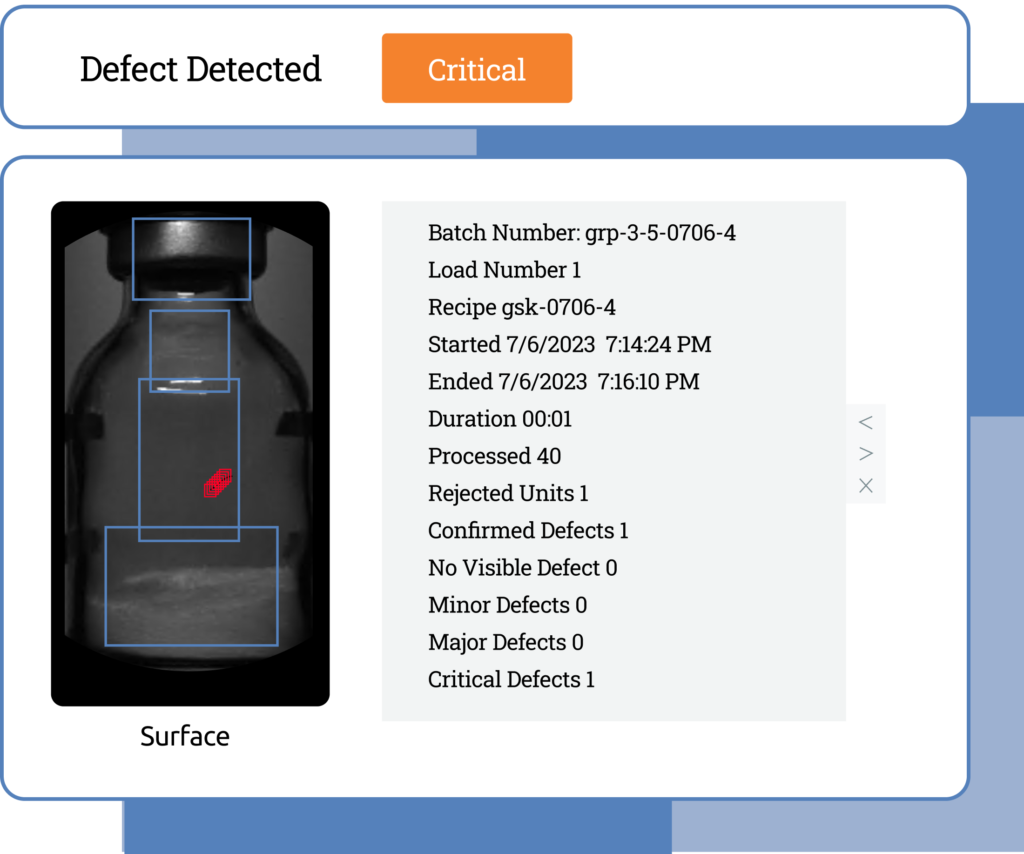